Welcome to Dilemmas of Meaning, a journal at the intersection of philosophy, culture, and technology. Here I ask a central question: Why do we use science-fiction analogies when talking about technology? It also highlights the gap between knowing of a difficult concept and actually understanding it, how corporations benefit from our confusion, and one way we can push back.
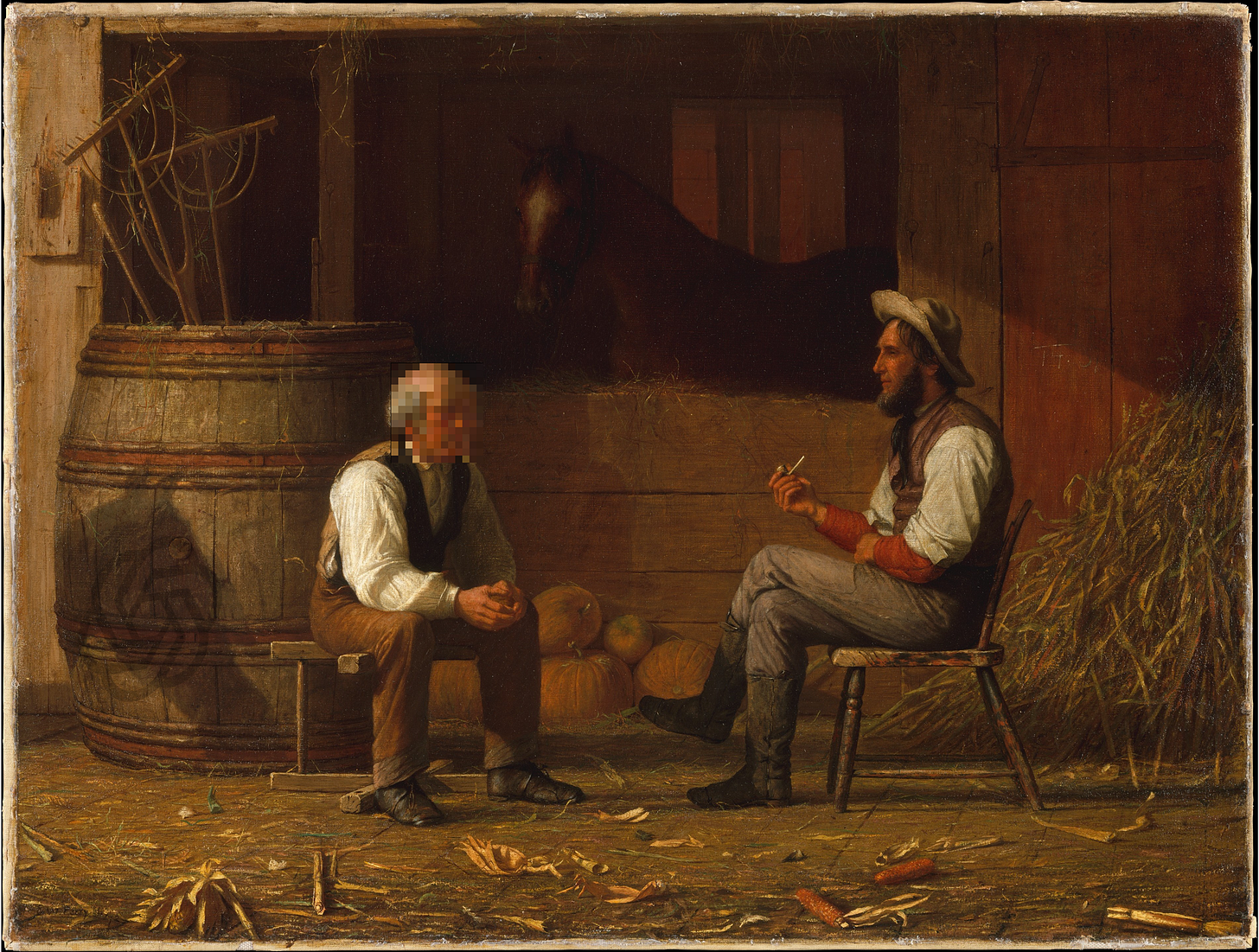
One thing shared by Crypto, Web3, and the Metaverse—other than having arrested the technological zeitgeist in the past 24 months—is that nobody knows what they mean. Well, apart from those championing these developments. The average person, however, understands mainly the mindshare these concepts command. All of us, whether we be experts, evangelists, or apathetic, are subject to public debates on regulations, policy, and their societal consequences. People feeling forced to speak about technology without expertise produces a way of talking about technological developments that impairs constructive public discourse and privileges powerful corporations.
Focusing on Artificial Intelligence (AI), a term so loaded with zeal it dominated the earnings calls for Meta, Microsoft, and Google. Decades of science-fiction and fantasy has primed the general audience as to what AI is supposed to look like. Without knowing how these systems work, these fictional examples make up our shared vocabulary. Open AI’s Chat-GPT has been compared to Her (2013), Hall 9000 , and Jarvis among others. These comparisons, built upon metaphors, shape the expectations, excitement, and scope of what technology developments might usher in: the future.
Why is this linking of AI, its fictional representations, and the future worrying? This confluence, I believe, constructs a dilemma of meaning that bisects public discourse on technological developments: 1) discourse based on the products as they are and 2) discourse on the products as we wish them to be. To unpack this dilemma, first a consideration of how metaphors are used. To be clear, this is not a polemic against metaphors generally, but a diagnosis of the effect using specifically fictional metaphors have and an outline of a potential remedy. So how do metaphors work?
When we use metaphors, we replace the primary term with a secondary term and its corresponding meaning. Take the example of Romeo calling Juliet the sun. Both terms, Juliet (the primary) and the sun (the secondary) exist independently of each other. Juliet is not the sun, and the sun is not Juliet. However, both contain a meaning that is, in the author’s mind, analogous; and, more than that, that meaning is better expressed in the secondary term. The sun it hot, warm, enriching. Crucially, to the reader, who is Juliet? We do not know. There is an inequality of information here. We know the sun but are relatively ignorant of Juliet. This inequality could be remedied by providing knowledge of Juliet; describing her in detail to leave the reader sure of a meaning behind the name. However, that is a lot of work for the author and not nearly as attractive to the audience. Therefore, by analogising the primary with the secondary, the author aims to transfer meaning to the former.
Recall, however, that despite this substitution, Juliet is still not the sun. Thus, there is a tension. To unravel this, we face it head on: what does Romeo (and thus the author) mean when he calls Juliet the sun? In answering that question, we engage in paraphrasing, for we (rarely) know what the author intended—so we make our own. Taking from the context, our associations with the secondary term (the sun), and the way the metaphor is used. Earlier, I gave my simple paraphrase (hot, warm, enriching), yours may be different. Indeed, yours may even vary from describing the sun independently. There may be emotions, images, ideas. By juxtaposing the sun and Juliet and forcing the reader to paraphrase, rather than constraining Juliet to the descriptions that might have been offered in the boring route, she is imbued with a meaning that is richer, varied, and spontaneous.
Yet, there remains something unique when using metaphors linked and localised to existing texts. This intertextual relationship fails to achieve the kind of layered paraphrasing as discussed above, resulting in shallow and one-dimensional meanings.
On Metaphors, Meaning, and the White Whale
If I call something my ‘white whale’, I’d wager Moby Dick does not form a strong part of the context that informs your paraphrasing. In fact, it is possible you have never read the tome. It has transcended Herman Melville’s magnum opus and entered the English-speaking lexicon. The context associated with the phrase ‘white whale’, therefore, lies in how it has been used since 1851. Conversely, let’s say I compare a product to R2-D2. While Star Wars has entered popular culture, the text of Star Wars has proved more rigid when it comes to the attached meaning. Thus, those familiar with R2-D2 paraphrase via the canon of the attached property; there is less room for the kind of nuance and spontaneity that might come from ‘white whale’ or even the sun. Curiously, this appears to be an effect hyperbolised in the medium of cinema and television; relatively recent and aggressively curated. I say this not to suggest that intertextuality is novel, it has existed long before James Joyce wrote Ulysses. Rather, due to the combination of a short timeframe and being intellectual properties tightly controlled by their owners, when we use recent fictional examples, their meaning remains calcified.
Understanding this then places the use of fictional secondary terms as metaphors in context. That their ability to function as rich secondary terms is not itself harmful, it is possible for one-note metaphors to form part of a rich vocabulary for discourse. However, what is harmful is that these examples constantly present a narrative that only supports the side of blind techno-optimism; benefiting the corporations who profit from this kind of hype. The narrative they present is one of technological developments as inevitable and progress. It exoticises them from reality. That narrative is an association with the future as better, cool, and inevitable.
The Future as Better, Cool, and Inevitable
Few things capture the imagination like the future; tomorrow we will run faster, stretch out our arms farther. By existing in that never-approaching reality, we can project upon it our hopes, dreams, and fears. This projection aids in seeing history as teleological, as a journey to somewhere, towards a destination filled primarily by speculation and emotion.
As a case study consider the narrative around Apple and AI. With the introduction of the Siri virtual assistant, comparisons were instantly drawn towards fictional computers based especially on its ability to understand and speak naturally. With this, it was heralded as ‘sheer magic’, a sea-change and a revolutionary computer interface that would upend all we previously knew. History has not been kind to those sentiments. How often do you use voice assistance? What was the future is now but an accidental button press. Conversely, Apple has added tools within their ecosystems that build upon machine learning to offer quality of life improvements in the background. Despite tools like your iPhone understanding when you’re searching for ‘cat’ photos and instantly organising your library to present your myriad feline photoshoots, there remain polemics decrying Apple falling behind in the AI race.
Crucially, this case study reveals two things. Firstly, absent technical expertise, the lack of good metaphors prevents public discourse amongst the many as shown by Apple’s quality of life focused developments. Secondly, and as with Siri, being equipped with a vocabulary sourced from fiction warps how technology developments are judged and seen. For if it aligns with what we might already be familiar with, it comes pre-packaged with the associated emotions; it has baggage. Divorced from the actual development they describe, a parallel technology is reified and continually reinforced by cherry picked examples. The consequence of this is confirmation bias run amok. When this fantastical idea of AI is met with a discussion that seeks to ground these ideals, such as policy proposals, regulation on AI, or with Siri a realistic assessment of quality, it is impossible to achieve any consensus. The facts at play are not shared, and those enraptured by the hype of these fictionalised products argue and posit based on that alone. This parallel world of hype benefits the corporations that own these AI products and grifters who ride waves of hype to swindle people.
Technical Gap and Language Games
Fundamentally, there is a technical information gap by which the lay public attempt (and fail) to overcome as they engage in discourse on technology. This gap is not limited to AI discourse considering the way social media algorithms have been talked about as unknown/dangerous/ethereal. If we hope to engage in constructive discussions about the effects of these technological developments, we—the general public— are woefully unequipped.
Taking this gap as their cause célèbre, a cadre of ‘demystifiers’ have arisen. You will find them all over the written world, aiming to present ‘accurate’ definitions of the technical underpinnings of these systems. In truth, their mission is fated to fail for they preach only to the converted. At best, they transmit a much-appreciated sentiment of scepticism, and at worse they remain a mere speed bump as they attempt to educate. Yet crucially, their problem is not one of knowledge but understanding. Let me explain with an example: Asking the average person how a smartphone camera works will rarely lead to an exploration of sensors and lenses and computational photography. Instead, I suggest we present examples or metaphors by which we understand cameras: mirrors, the eye, photoshop. These examples are not themselves wholly accurate, but by using these examples and trading them around — like a tennis rally — we hope to transmit an understanding. A is for Apple/A is for Ant. By sharing these examples, we may not, if not acquire knowledge of precisely what a camera is or what the letter A is, become able to use it in practice. By dispensing with achieving knowledge as the only solution, I believe we achieve understanding.
Framing understanding in this way recalls the philosophical conception of language as a game.
For Ludwig Wittgenstein, language and, importantly, meaning, lies not in a definition but in use. In this way, meaning is contained neither in some external factor to the word in question nor a representation of a picture or concept in the user’s mind. In searching for meaning, we are instructed to look rather than think. This means rather than deriving generalising definitions we jump down the proverbial rabbit hole and appreciate the different ways words are used. Evidence rather than theory. How, you might ask, are we ever to cope if meaning is rooted in such a fluid practice as use? We consider language itself as a kind of game. Just as we cannot provide a decisive definition of ‘game’ (is chess a game, what about debating) we cannot agree on what is common to all these activities and present that as a meaning. Therefore, instead of finding the core, we find ‘family resemblances’: exploring the word’s uses through “a complicated network of similarities overlapping and criss-crossing.”
Appreciating this explains why the discourse around AI tools functions as its own language game. Rather than understanding the core or definition of these tools, we trade examples. AI is used in this way, and that way, and this way, functioning as a linguistic rally. Reconsidering language and meaning in this way explains why technology discourse still relies on fictional examples, despite their being rigid and inaccurate. That’s what discourse is, a trading of examples back and forth, a type of language game.
While knowledge might be ideal, the belief that as technology becomes increasingly complex it remains possible to share an accurate and technical knowledge of these developments is fanciful. It just doesn’t scale. By focusing on understanding and especially judging understanding by how the word is used in practice, I believe we meet people where they are. As discussed above, people already use examples, why limit it to fictional examples and their inaccurate, hype promoting by-product. Why can’t we provide better, more critical examples?
Examples of Examples
Exemplary of this turn from knowledge to understanding, is the recent New Yorker essay by Ted Chiang. Not only does Chiang write with grace and poise, but he also anchors his analysis of Chat-GPT on an example. Rather than directly defining the product on a definition of the capabilities, limitations, and dangers of Chat-GPT, he provides a series of examples, with each one building on another: fuzzy images, low bitrate mp3s, lossy compression, Xerox photocopiers. The article takes each example in turn, increasing in complexity until it reaches the point Chiang wishes to make “that the photocopier was producing … copies that seem accurate when they weren’t.” He then transitions from this example to finally talking about Chat-GPT, at this point the reader has been introduced to the words that make up the language game he’s playing (blurry, fuzzy images, compression, photocopiers, jpegs etc) and having used them, they are given meaning. We’re in his world; and, while we might not have full knowledge of all those terms, within the context of his critique of Chat-GPT we understand where and how he is getting there. I leave off my analysis here and encourage you to read the piece. The key here is Chiang’s rejection of the normal format of AI demystifiers; he is not providing a technical (and accurate) definition of AI tools and products and then engaging in a lecture. Discourse is not a lecture, it’s a game.
Discourse is not a Lecture, it’s a Game
I want to finish by considering this proposition and why I think it is important to take to heart for those that are critical of the cost of tech overreach. By virtue of the overwhelming capital advantage, the tech industry will seek to bend narrative in beneficial ways, providing only the examples that allow their products to mean what they want them to mean. Reality be damned. I have focused on fictional examples and how their rigid structure restrains public discourse in ways that can impair critique. Seeing Siri as the future made it difficult to consider that it actually wasn’t that useful for our lives. If it’s the future, its usefulness is implied. Another fictional example that lay outside the scope of this essay is AI doomerism, which functions as another quasi-fictional example albeit more pessimistic than the image of the future as cool and exciting. Perhaps I will return to unpack that metaphor. If we are to hold power accountable, we cannot run from the game and lecture from the side lines we must join the rally. Tech has served and now we must return.